Unlocking AI’s Potential in Computational Science Without Compromising Reliability and Precision
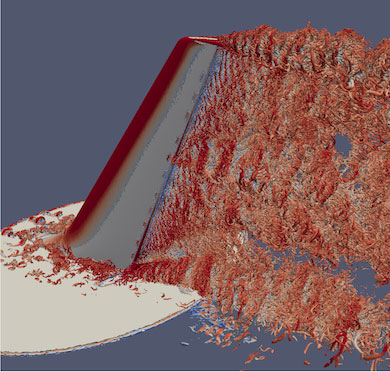
High-performance computing (HPC) is the perfect platform for AI and machine learning (AI/ML) research. This is because modern leadership class supercomputers rely on the massive parallelism of GPUs to perform scientific computation at exascale. Those same GPUs are the bedrock that the proliferation of AI/ML technologies are built upon. Therefore, these machines make it possible to combine traditional simulation workflows with AI/ML techniques and offer the potential to significantly accelerate scientific discovery through the use of partial to full surrogate models. It is essential for the community to fully unlock this potential in order to fully leverage modern supercomputers.
Exciting discoveries at an accelerated pace
We are seeing the adoption of AI/ML tools at a rapid pace with exciting results. Researchers are leveraging AI/ML across the scientific computing spectrum, from battery research to weather forecasting, and employing new workflows and mathematical models, including physics-informed neural networks and in situ co-processing.
For example, AI-driven weather forecasting programs like FourCastNet exceed the accuracy of modern weather forecasting for small-scale variables such as local precipitation. FourCastNet produces week-long forecasts in less than two seconds. These tools have exciting implications for wind energy planning and extreme weather prediction.
In a collaboration between Pacific Northwest National Laboratory and Microsoft, material scientists and computational chemists are leveraging AI tools to discover new battery materials at an incredible rate. They employed powerful new computational chemistry surrogate models running on HPC systems to propose 32 million candidate battery materials, and then select down to just 18 promising candidates in a matter of just 80 hours. This unprecedented workflow has taken a typically years-long search for a new stable battery material down to a matter of weeks.
Within Kitware, we are integrating AI/ML research into our Catalyst platform to enable users to enhance their scientific simulation code with modern AI/ML capabilities.
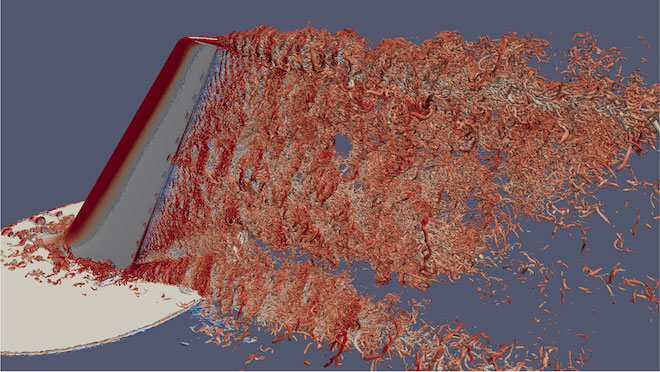
Catalyst is bridging the gap between simulation and AI
Across the computational science field, AI is reshaping the landscape of simulation-based research. However, in spite of their demonstrated potential, roadblocks have slowed the adoption of AI/ML in mature scientific simulations.
Traditional simulation tools, fine-tuned over decades, are complex and domain-specific. Researchers have expressed hesitation to integrate AI into these systems due to concerns about “black box” models that lack transparency or fail to deliver consistent, measurable benefits. This is where many current AI/ML applications fall short in scientific fields. The question is: How can we unlock the potential of AI/ML in computational science without disrupting the reliability and precision that researchers require?
We see the answer in creating tailored AI/ML integrations that work hand-in-hand with existing simulation codes. We are using Catalyst to develop an accessible, in situ machine learning toolbox that will enhance the performance of well-established scientific simulations. Scientists will be able to reap the benefits of AI/ML without sacrificing accuracy or control. This toolbox would allow simulation practitioners and developers to explore bespoke AI/ML solutions that can augment their simulation, without resorting to expensive and intrusive code integration.
The potential of this technology is not just short-term, but it could transform how scientists conduct simulations long-term. By reducing the barriers to AI adoption, this research is investing in a future where cutting-edge tools are more accessible, adaptable, and impactful. Scientists will no longer have to choose between established, reliable methods and the innovation of AI. Rather, they will have the opportunity to leverage AI that enhances their simulation capabilities while maintaining rigorous standards of scientific inquiry.
If you would like to learn more about accelerating AI integration into your HPC research, contact our team. We can work with you to bring your visualization pipelines directly to simulation environments, reducing IO costs and accelerating time-to-insight.