MHSRS 2024
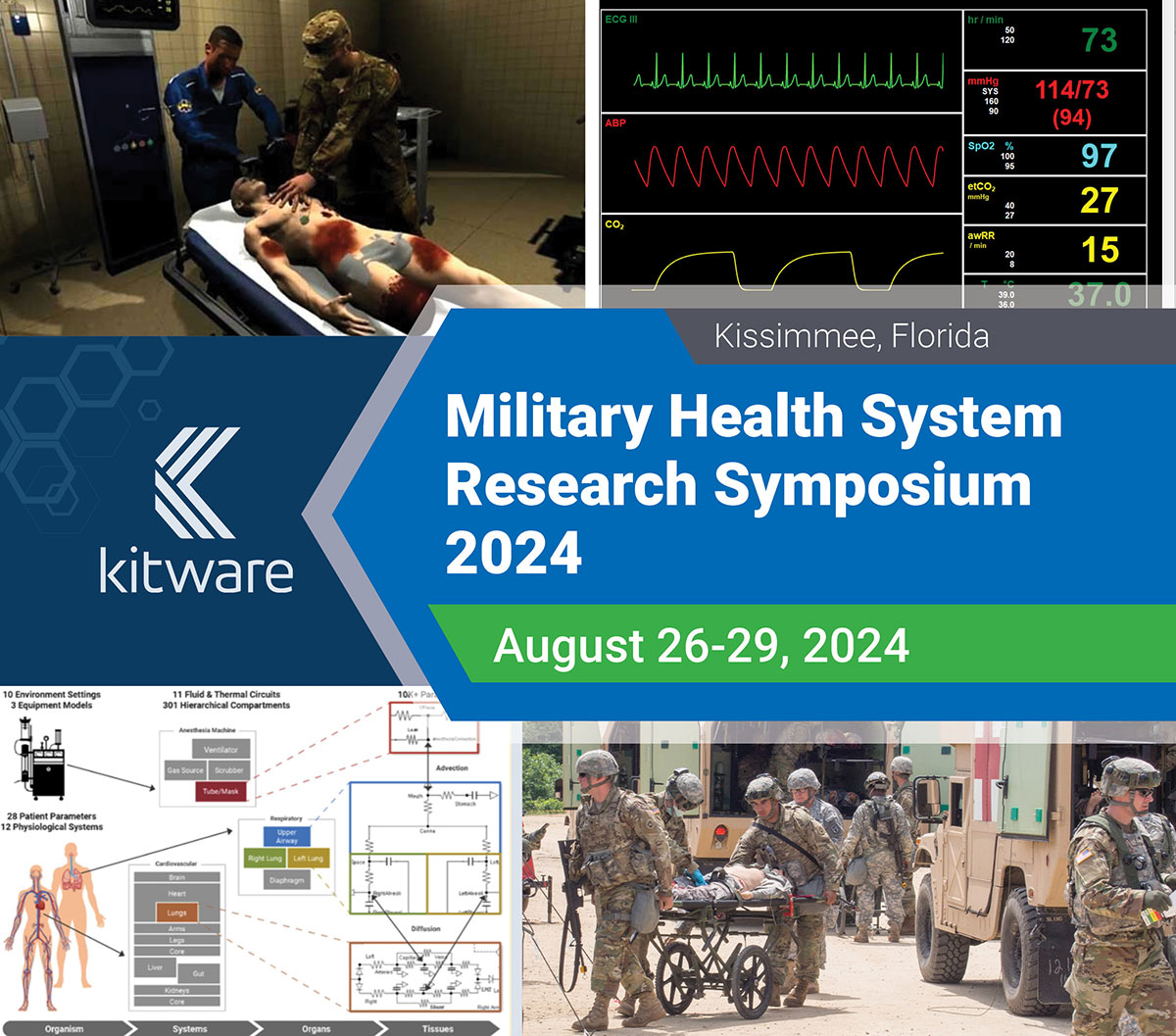
August 26-29, 2024 in Kissimmee, Florida
The Military Health System Research Symposium (MHSRS) is the leading scientific conference organized by the Department of Defense, dedicated to addressing the specific medical requirements of the military. MHSRS promotes collaboration among military healthcare professionals, academic institutions, and industry experts in the field of medical and healthcare research. The symposium’s agenda includes topics such as combat injury care, operational medicine for military personnel, clinical and rehabilitative medicine, medical simulation and information technology, military infectious diseases, and the effects of radiation on health.
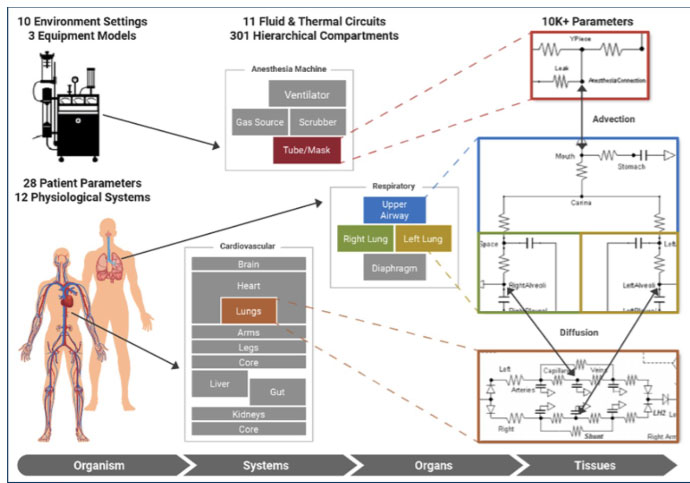
This is Kitware’s second year participating in this event. We are excited to be exhibiting again this year to showcase our innovative medical and biomedical software. We will also be sharing the details of our involvement in the DARPA ITM and DARPA Triage programs, both of which leveraged the Pulse Physiology Engine. Pulse was used to augment real world data with synthetic physiology data for medical machine learning tasks, making resource and time-limited medical triage decisions and predicting the need for life-saving interventions.
We are also proud to share our analysis of spine shape in positional MRI (pMRI) scans to predict lower back pain (the most commonly reported musculoskeletal injury across the U.S. Armed Forces). We hope to extend this work to provide a preventative personal risk assessment of injury for the warfighter.
To learn more about our capabilities, visit us at booth 313 or contact our team.
Kitware’s Activities and Involvement
An artificial intelligence solution to human-trusted medical triage decision making
Paper
Authors: Aaron Bray, Emily Veenhuis, Brian Hu, Ph.D., David Joy, Bill Ray, Jeffery B. Webb, Rachel B. Clipp, Ph.D., and Arslan Basharat, Ph.D.
AI is proposed as a solution to assist humans with making difficult medical triage decisions. The ALIGN system uses Large Language Models (LLMs) to reason over all possible choices flexibly weighing different attributes and generating a natural language explanation of its decisions. However, it is unclear how LLMs perform in the medical triage domain, where decision-making must take into account both basic medical knowledge and other human attributes. Therefore, there is a critical need for a dataset of casualties with tags (Immediate, Expectant, Delayed, or Minimal) that can be used to assess LLM performance.
We developed a synthetic data set using the open source Pulse Physiology Engine. Pulse is a computational physiology engine that uses physics-based models to dynamically calculate the vital signs for patients during healthy, disease, injury, and treatment states. Male and female synthetic patients were given varying severities of injuries, including tension pneumothorax, hemorrhage, and airway trauma (alone and in combination). Each patient injury combination was simulated for 1 hour to represent a timeline of the initial assessment of the injuries through potential medical intervention. For each patient, quantitative (e.g., heart rate, blood pressure, and respiration rate) and qualitative (e.g., ambulatory, consciousness, and AVPU) observations were output every 3 minutes after the injuries were applied. Retrieval-Augmented Generation (RAG) was used to train the LLM on the START tagging protocol. A natural language description of the patient, injuries, and observations using the START protocol terminology was written. These were provided to the LLM to test the accuracy of the tagging predictions compared to the ground truth tags.
This work has resulted in a large open dataset of approximately 4,000 descriptions and their expected tag. The initial LLM tag predictions had an accuracy of 63% compared to the ground truth tags.
This multilayered dataset will be released publicly via the Pulse Physiology Engine suite. Its utility is clear through its use across multiple medical triage decision support projects. Future work includes augmentation of the dataset with additional injuries and tagging protocols (SALT, BCD Sieve) and use of the dataset to further train AI algorithms for decision support.
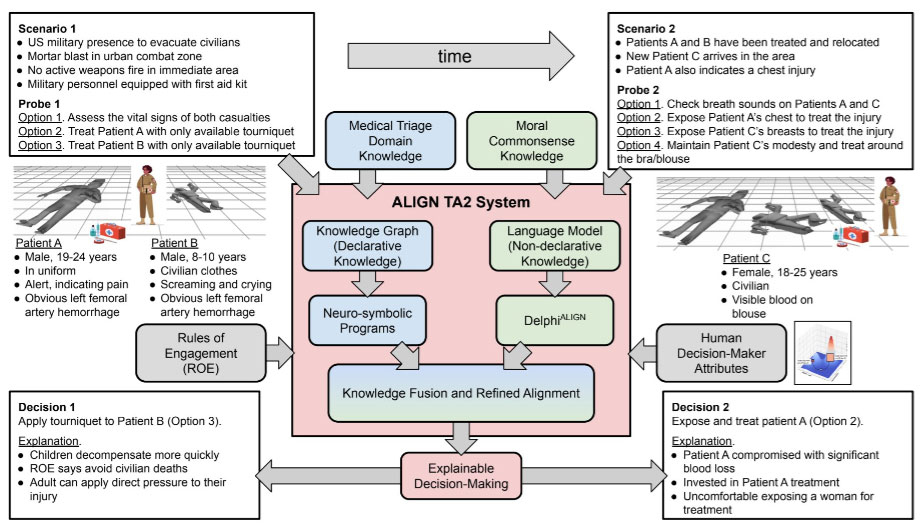
Predicting the need for life-saving interventions during triage using a deep time-series predictor pipeline
Paper
Authors: Aaron Bray, Jared Vicory, Ph.D., Matthew Bernstein, Emily Veenhuis, Barry Ravichandran, Bob Marinier, Ph.D., Alyssa Tanaka, Ph.D., and Rachel B. Clipp, Ph.D.
In mass casualty scenarios it can be difficult to prioritize life-saving interventions to the most critically injured. Providers may be monitoring a large number of patients with injuries that could cause rapid destabilization. However, early treatment of injuries, particularly hemorrhage, prior to patient decompensation have been shown to yield improved outcomes. Therefore, autonomous monitoring and identification of the need for life-saving intervention is a significant need, particularly in resource limited circumstances.
We are combining deep learning with a symbolic predictor to identify the need for a life-saving intervention prior to patient decompensation. The system is being trained using a combination of patient data and synthetically generated data. The open source Pulse Physiology Engine was used to create a synthetic injury data set that included tension pneumothorax, hemorrhage, and airway trauma. These injuries were applied with varying severities in isolation and in various combinations. We are also using the MIMIC-III and -IV clinical datasets on Physionet and data collected as part of the DARPA RITMO project. We have developed a machine learning pipeline to input the synthetic data and train a temporal fusion transformer model to identify patient decompensation. In parallel, the ISAAC symbolic predictor intakes patient information and vital sign data and makes an injury prediction and treatment recommendation. ISAAC encodes “textbook” information that is applied to patient time-series vitals data, demographics, and clinician observations to provide a treatment recommendation.
Our preliminary pipeline was executed on a subset of synthetic data for hemorrhage with the goal of predicting patient decompensation. Patient decompensation (cardiovascular collapse) was predicted with 76% accuracy and with good temporal accuracy, a mean and standard deviation of 25.3s and 107.1s within the 100 min prediction period.
Our preliminary pipeline shows significant promise to identify the need for life-saving interventions prior to patient decompensation. Our next steps are to expand the deep-series time predictor to other injuries (pneumothorax and airway trauma), use the ISAAC prediction as an input to the deep-series time predictor, and further train and validate the pipeline using the patient data and the synthetic data.
Personalized Evaluation of the lumbar spine for susceptibility to pain and injury in warfighters
Paper
Authors: Beatriz Paniagua, Ph.D., Ye Han, Ph.D., James Fishbaugh, Ph.D., Brad Moore, Ph.D., Simon Tang, Ph.D.
Musculoskeletal injuries are the cause for 60% of limit-duty days and 65% of non-deployments in soldiers. Lower back pain (LBP) is reported as the most common injury site across the U.S. Armed Forces, whether deployed or at home station. The clinical assessment of spinal structures, such as the intervertebral disc (IVD), rely on the qualitative, subjective evaluation of MRI, and this has typically corresponded poorly with the pain presentation. To bridge this diagnostic gap, we propose to use advanced non-Euclidean statistical shape analysis methods to define pathoanatomical biomarkers in individuals who are susceptible to load-induced LBP. We present this study as a proof-of-concept for injury prevention intended to decrease limited duty days, increase deployability rates, and decrease medical separation rates. These biomarkers will also serve as therapeutic targets for treating LBP in warfighters.
For this study, we obtained 11 positional MRI (pMRI) 1 sequential scans from civilian participants (4M/7F), between 18 and 30 years old with a BMI lower than 30 kg/m 2 . For 2 hours, pMRIs and self-reported pain (visual analogue scale) were collected every 15 minutes. Each sagittal slice in the scan was evaluated and the IVD contour was defined for each lumbar segment. We then performed geodesic logistic regression (GLR) 2 to each set of sequential pMRI scans for each subject, to relate pain symptoms to IVD shape features.
Our GLR framework results indicate that there is a clear separation between pain / no pain based on the observed IVD shapes while prolonged standing in an individual. Note that the applied GLR model is different from other classification methods like support vector machine that tries to maximize the separation margin between different data points (e.g. pain/no pain). Instead, the parameter estimation process would generate a GLR model that minimizes the overall negative log-likelihood function. Our GLR results show that we find almost perfect cut- offs for most of the subjects. This indicates the feasibility of identifying individuals who may suffer from LBP solely based on their spinal anatomy.
This framework sets the basis for the design of a computer-assisted injury prevention system, that will offer army physicians a valuable tool to quantitatively evaluate the propensity of a certain soldier to suffer LBP during deployment. The proposed work will ultimately prevent and reduce soldier suffering.
Medical and Biomedical Systems at Kitware
Kitware’s interdisciplinary team of computer scientists, software engineers, and imaging experts has extensive expertise in medical imaging AI. Our technical areas of focus include:
- Bioinformatics
- Computational physiological modeling
- Dental, craniomaxillofacial, and musculoskeletal image analysis
- Dataset collection and annotation
- Digital pathology
- Image-guided intervention and surgical planning
- Medical image analysis
- Medical simulation
- Medical visualization
- Surgical robotics
- Ultrasound systems
Kitware provides collaborative research, development, and technology integration services for government, academic research partners, and commercial customers in the medical and biomedical business sectors. Our team understands the critical need for robust, reliable, and high-performance software support in developing and deploying AI solutions in medical applications. We innovate new methodologies, generate prototypes for proof of concept and feasibility study, or participate in full product development for FDA approval.
Our solutions come with unlimited rights to the U.S. government, including no licensing fees or restrictions. For commercial customers, you own the proprietary source code we develop and the intellectual property. We can provide support at any stage of the medical product lifecycle, from initial R&D to IEC 62304 and HIPAA-compliant product development.
Kitware’s development, testing, and documentation practices are aligned with FDA requirements and HIPAA technical safeguards for software products. Kitware can sign NDAs and HIPAA BAAs, and we have the infrastructure to handle projects subject to CUI, EC, ITAR, and HIPAA regulations.
Kitware can help you harness the power of open source technology to develop cutting-edge medical software efficiently. Contact our team to learn more.