The Geospatial Intelligence Symposium (GEOINT)
The GEOINT Symposium is one of the largest gatherings of geospatial intelligence professionals in the U.S., with attendees from government, industry, and academia. Kitware has been participating extensively at GEOINT for several years now and is a highly involved member of the geospatial intelligence community. We work closely with the intelligence community and government defense agencies on geospatial intelligence R&D projects that require experts in AI, machine learning, and computer vision. In addition to exhibiting this year (find us at booth #1412), we are presenting two training sessions and three lightning talks. If you would like to discuss collaboration opportunities, please email our team at computervision@kitware.com.
Would you like to discuss collaboration opportunities?
Events Schedule
Towards Ethical AI and Trusted Autonomy: Legal, Moral, and Ethical Considerations from the DARPA URSA Program
Presenter: Anthony Hoogs, Ph.D., Vice President of Artificial Intelligence at Kitware
Kitware values the need to understand the ethical concerns, impacts, and risks of using AI. Urban Reconnaissance through Supervised Autonomy (URSA) is a DARPA program with the goal of developing autonomous systems that work with humans to provide actionable intelligence in complex urban environments. URSA involves interactions with non-combatant civilians, and is exploring legal, moral, and ethical (LME) considerations of operating autonomous systems in gray zone scenarios. URSA has formulated the concept of DevEthicalOps (Ethical Development and Operations), or incorporating ethical considerations into all aspects of system design, development, execution, and data management. This talk will present two LME-specific features that we have implemented in the URSA system to prevent the collection of sensitive information and harm of sensitive areas (e.g. places of worship). These LME additions have improved the performance of the baseline system, suggesting that ethical constraints may provide important benefits in addition to assuring the ethical and trusted use of the system.
Disinformation in the GEOINT Domain: Threats, Challenges, and Defenses
Presenter: Arslan Basharat, Ph.D., Assistant Director of Computer Vision at Kitware
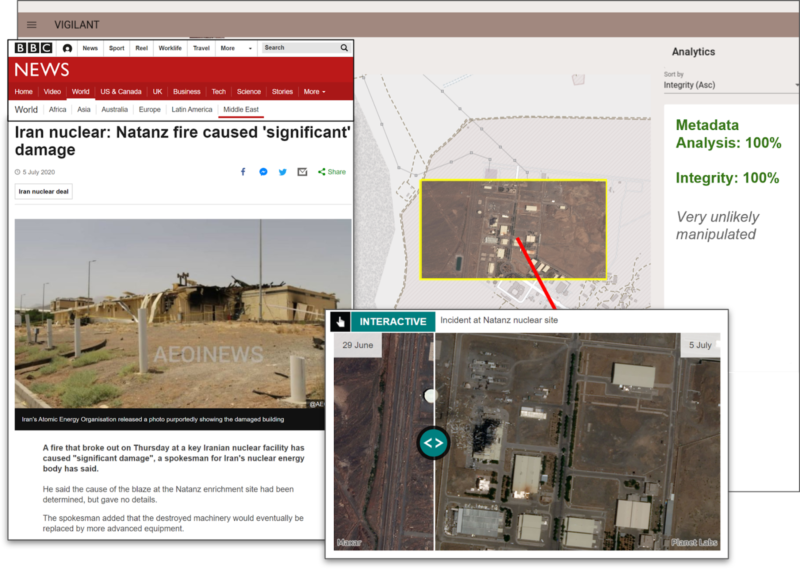
As media generation and manipulation technologies are advancing rapidly, Federal R&D programs such as DARPA MediFor and SemaFor have been instrumental in advancing forensic defenses that incorporate artificial intelligence, machine learning, computer vision, and natural language processing. This training session will describe and illustrate the prevalent threats posed by state-of-the-art automated media generation and manipulation technologies, and demonstrate the common challenges faced by ML approaches for detecting and analyzing such artifacts. Arslan will discuss how multimodal and semantic analytics can address these challenges through state-of-the-art approaches for detecting, attributing, and characterizing disinformation that involves image and text modalities, through case studies in news articles. The training session will also introduce the image forensics capabilities in the VIGILANT satellite imagery exploitation tool (developed by Kitware with funding from AFRL and NGA), which allows a GEOINT analyst to detect, localize, and analyze pixel manipulations in satellite images of questionable authenticity. [Sign up for this training session]
Novelty Detection to Discover Salient Unknown Unknowns
Presenter: Anthony Hoogs, Ph.D., Vice President of Artificial Intelligence at Kitware
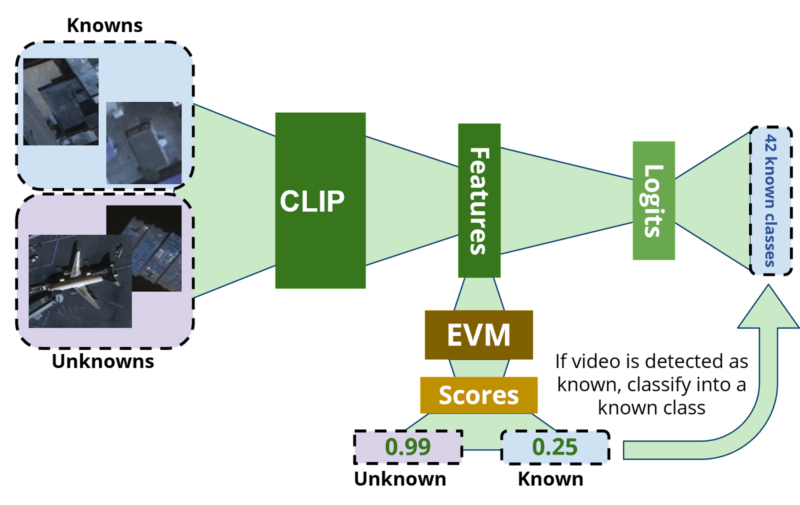
As a result of deep learning approaches, revolutionary advances have been achieved in object detection and classification in satellite imagery. Traditional approaches follow the standard target recognition paradigm by assuming a fixed set of known object classes (e.g. types of airplanes), but these methods can miss important targets that are not sufficiently similar to known classesl. In this talk, Anthony will cover novelty detection, which is an emerging technique to explicitly detect unknown objects, while continuing to correctly classify instances of known object types. Kitware has developed a method that uses extreme value theory and has conducted experiments on the xView dataset. Our results indicate that our approach is more effective at finding novel objects, even when novel classes are similar to known ones, than the baseline approach of thresholding on classification probabilities of known classes. This work is a collaboration with the University of Colorado and Notre Dame.
Explainable AI for Imagery Analysis
Presenter: Anthony Hoogs, Ph.D., Vice President of Artificial Intelligence at Kitware
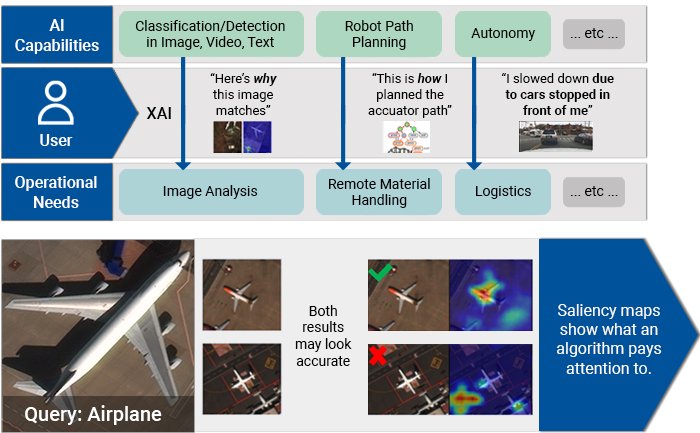
It is important for analysts to have a sense of how data and metadata conditions affect a model’s performance so they can develop a justified, calibrated sense of trust in AI model. In this training session, you will learn how contemporary deep learning networks are used in object detection and image retrieval algorithms, and how techniques for Explainable Artificial Intelligence (XAI) can help analysts develop a sense of not just “what result did the AI compute?” but “why did the AI compute that result?” Anthony will explain how the developing field of XAI applies to typical image analysis workflows, focusing on object detection and image retrieval as case studies. He will also review the latest in XAI, emphasizing how visual saliency maps can illuminate the behavior of deep learning models, and demonstrating how analysts can use XAI to understand when and why deep-learning models do (and don’t) perform as expected. [Sign up for this training session]
Kitware Results on Phase 1 of the IARPA Smart Program
Presenter: Matt Leotta, Ph.D., Assistant Director of Computer Vision at Kitware
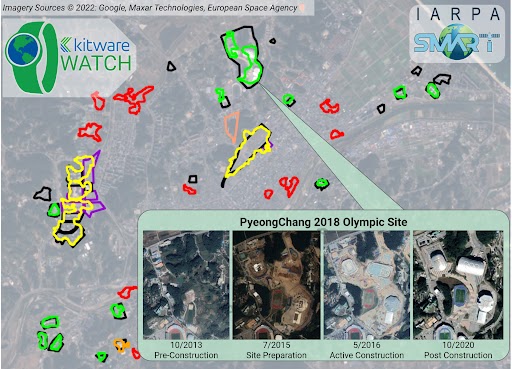
The IARPA SMART program is bringing together many government agencies, companies, and universities to research new methods and build a system to search through enormous catalogs of satellite images from various sources to find and characterize relevant change events as they evolve over time. Kitware is leading one of the performer teams on this program, which includes DZYNE Technologies, BeamIO, Inc., Rutgers University, the University of Kentucky, the University of Maryland at College Park, and the University of Connecticut. At GEOINT 2021, Kitware summarized our preliminary results on this program and introduced our system called WATCH (Wide Area Terrestrial Change Hypercube). This year, we will summarize our new progress during the first eighteen-month phase of the program, and show improvements in detecting new construction and classifying construction phases. We will also highlight our research results, system integration/deployment progress, and developed open source tools which are already available free for community use.
Kitware’s Computer Vision Focus Areas
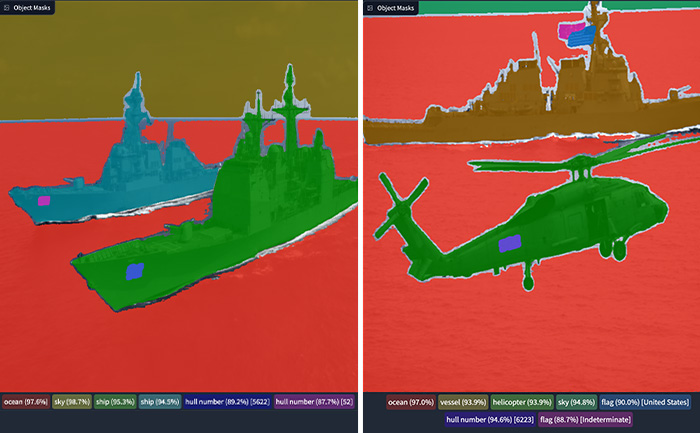
Generative AI
Through our extensive experience in AI and our early adoption of deep learning, we have made significant contributions to object detection, recognition, tracking, activity detection, semantic segmentation, and content-based retrieval for computer vision. With recent shifts in the field from predictive AI to generative AI (or genAI), we are leveraging new technologies such as large language models (LLMs) and multi-modal foundation models that operate on both visual and textual inputs. On the DARPA ITM program, we have developed sample-efficient methods to adapt LLMs for human-aligned decision-making in the medical triage domain.
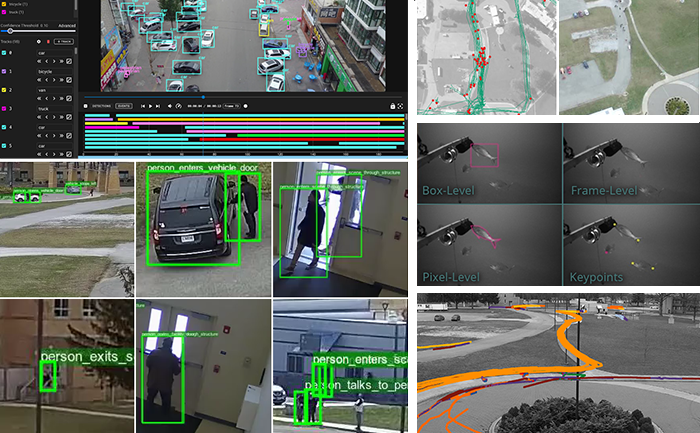
Dataset Collection and Annotation
The growth in deep learning has increased the demand for quality, labeled datasets needed to train models and algorithms. The power of these models and algorithms greatly depends on the quality of the training data available. Kitware has developed and cultivated dataset collection, annotation, and curation processes to build powerful capabilities that are unbiased and accurate, and not riddled with errors or false positives. Kitware can collect and source datasets and design custom annotation pipelines. We can annotate image, video, text and other data types using our in-house, professional annotators, some of whom have security clearances, or leverage third-party annotation resources when appropriate. Kitware also performs quality assurance that is driven by rigorous metrics to highlight when returns are diminishing. All of this data is managed by Kitware for continued use to the benefit of our customers, projects, and teams. Data collected or curated by Kitware includes the MEVA activity and MEVID person re-identification datasets, the VIRAT activity dataset, and the DARPA Invisible Headlights off-road autonomous vehicle navigation dataset.
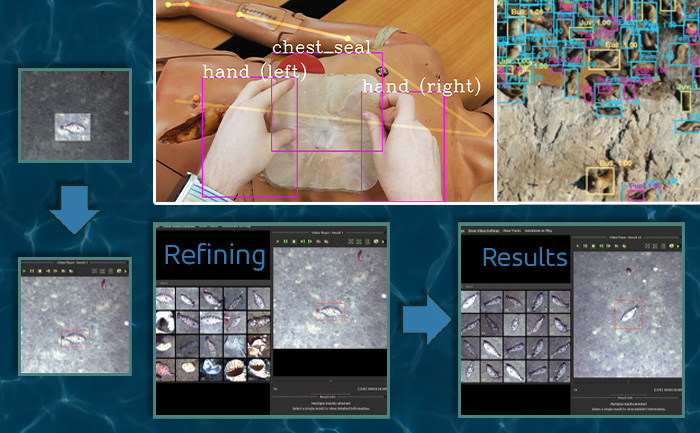
Interactive Artificial Intelligence and Human-Machine Teaming
DIY AI enables end users – analysts, operators, engineers – to rapidly build, test, and deploy novel AI solutions without having expertise in machine learning or even computer programming. Using Kitware’s interactive DIY AI toolkits, you can easily and efficiently train object classifiers using interactive query refinement, without drawing any bounding boxes. You are able to interactively improve existing capabilities to work optimally on your data for tasks such as object tracking, object detection, and event detection. Our toolkits also allow you to perform customized, highly specific searches of large image and video archives powered by cutting-edge AI methods. Currently, our DIY AI toolkits, such as VIAME, are used by scientists to analyze environmental images and video. Our defense-related versions are being used to address multiple domains and are provided with unlimited rights to the government. These toolkits enable long-term operational capabilities even as methods and technology evolve over time.
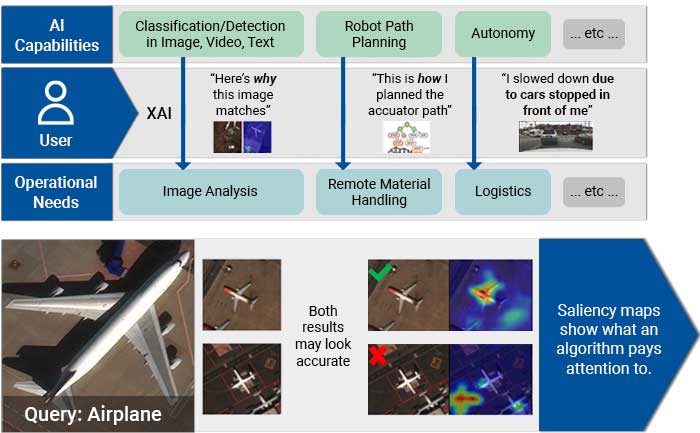
Explainable and Ethical AI
Integrating AI via human-machine teaming can greatly improve capabilities and competence as long as the team has a solid foundation of trust. To trust your AI partner, you must understand how the technology makes decisions and feel confident in those decisions. Kitware has developed powerful tools, such as the Explainable AI Toolkit (XAITK), to explore, quantify, and monitor the behavior of deep learning systems. Our team is also making deep neural networks more robust when faced with previously-unknown conditions, by leveraging AI test and evaluation (T&E) tools such as the Natural Robustness Toolkit (NRTK). In addition, our team is stepping outside of classic AI systems to address domain independent novelty identification, characterization, and adaptation to be able to acknowledge the introduction of unknowns. We also value the need to understand the ethical concerns, impacts, and risks of using AI. That’s why Kitware is developing methods to understand, formulate and test ethical reasoning algorithms for semi-autonomous applications. Kitware is proud to be part of the AISIC, a U.S. Department of Commerce Consortium dedicated to advancing the development and deployment of safe, trustworthy AI.
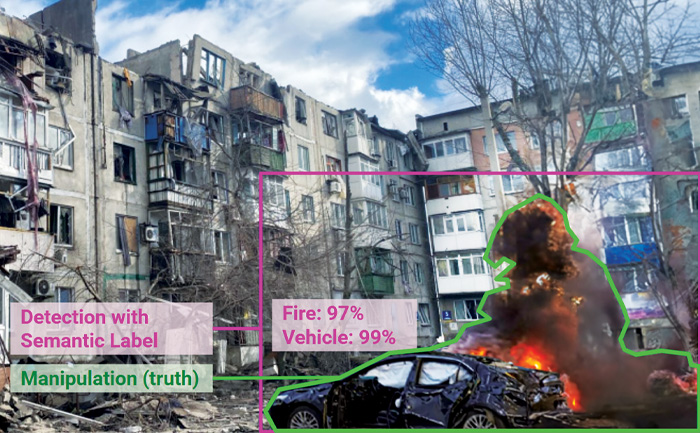
Combatting Disinformation
In the age of disinformation, it has become critical to validate the integrity and veracity of images, video, audio, and text sources. For instance, as photo-manipulation and photo-generation techniques are evolving rapidly, we continuously develop algorithms to detect, attribute, and characterize disinformation that can operate at scale on large data archives. These advanced AI algorithms allow us to detect inserted, removed, or altered objects, distinguish deep fakes from real images or videos, and identify deleted or inserted frames in videos in a way that exceeds human performance. We continue to extend this work through multiple government programs to detect manipulations in falsified media exploiting text, audio, images, and video.
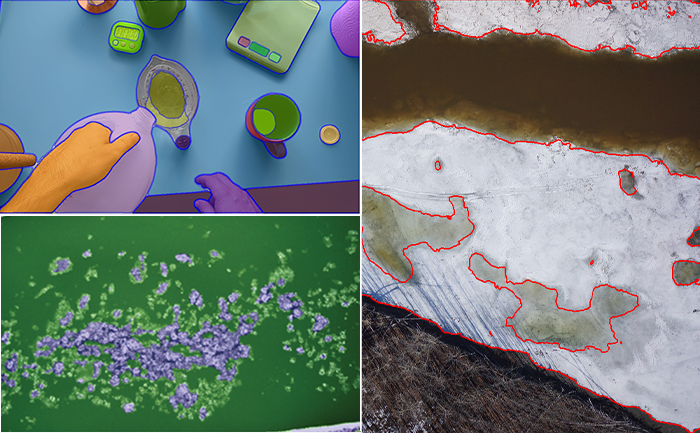
Semantic Segmentation
Kitware’s knowledge-driven scene understanding capabilities use deep learning techniques to accurately segment scenes into object types. In video, our unique approach defines objects by behavior, rather than appearance, so we can identify areas with similar behaviors. Through observing mover activity, our capabilities can segment a scene into functional object categories that may not be distinguishable by appearance alone. These capabilities are unsupervised so they automatically learn new functional categories without any manual annotations. Semantic scene understanding improves downstream capabilities such as threat detection, anomaly detection, change detection, 3D reconstruction, and more.
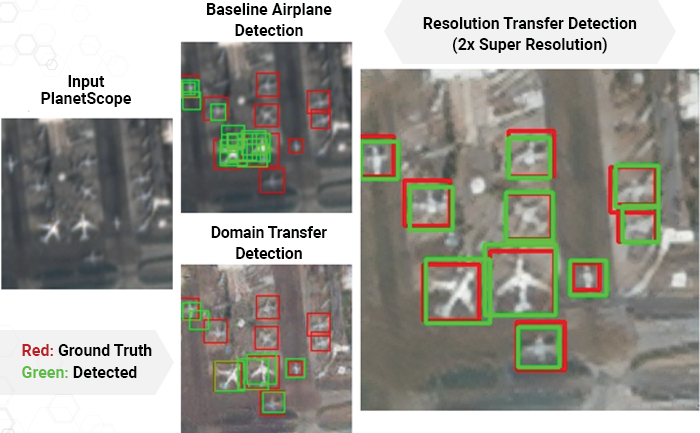
Super Resolution and Enhancement
Images and videos often come with unintended degradation – lens blur, sensor noise, environmental haze, compression artifacts, etc., or sometimes the relevant details are just beyond the resolution of the imagery. Kitware’s super-resolution techniques enhance single or multiple images to produce higher-resolution, improved images. We use novel methods to compensate for widely spaced views and illumination changes in overhead imagery, particulates and light attenuation in underwater imagery, and other challenges in a variety of domains. Our experience includes both powerful generative AI methods and simpler data-driven methods that avoid hallucination. The resulting higher-quality images enhance detail, enable advanced exploitation, and improve downstream automated analytics, such as object detection and classification.
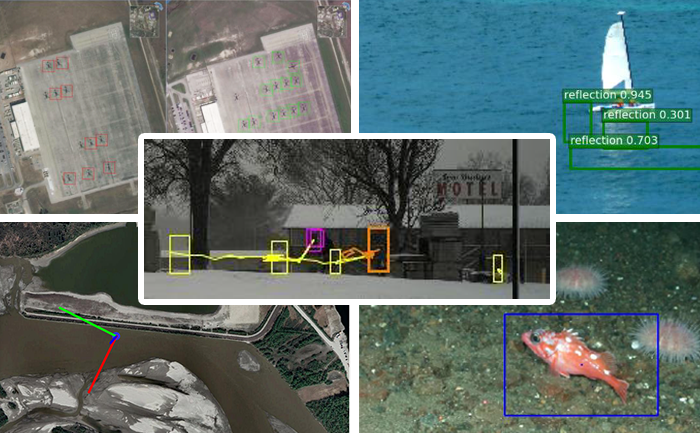
Object Detection and Classification
Our video object detection and tracking tools are the culmination of years of continuous government investment. Deployed operationally in various domains, our mature suite of trackers can identify and track moving objects in many types of intelligence, surveillance, and reconnaissance data (ISR), including video from ground cameras, aerial platforms, underwater vehicles, robots, and satellites. These tools are able to perform in challenging settings and address difficult factors, such as low contrast, low resolution, moving cameras, occlusions, shadows, and high traffic density, through multi-frame track initialization, track linking, reverse-time tracking, recurrent neural networks, and other techniques. Our trackers can perform difficult tasks including ground camera tracking in congested scenes, real-time multi-target tracking in full-field WAMI and OPIR, and tracking people in far-field, non-cooperative scenarios.
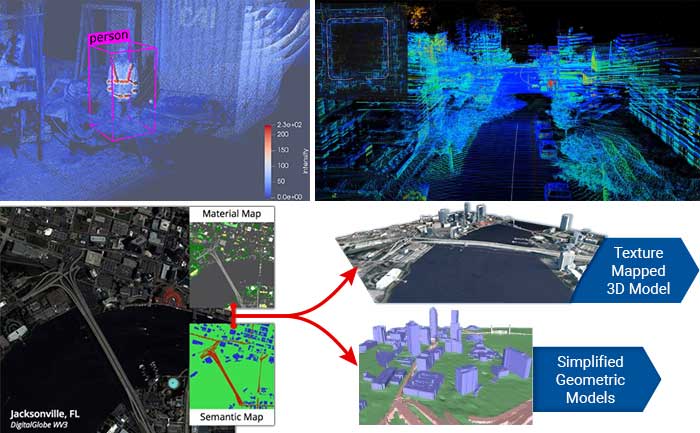
3D Reconstruction, Point Clouds, and Odometry
Kitware’s algorithms can extract 3D point clouds and surface meshes from video or images, without metadata or calibration information, or exploiting it when available. Operating on these 3D datasets or others from LiDAR and other depth sensors, our methods estimate scene semantics and 3D reconstruction jointly to maximize the accuracy of object classification, visual odometry, and 3D shape. Our open source 3D reconstruction toolkit, TeleSculptor, is continuously evolving to incorporate advancements to automatically analyze, visualize, and make measurements from images and video. LiDARView, another open source toolkit developed specifically for LiDAR data, performs 3D point cloud visualization and analysis in order to fuse data, techniques, and algorithms to produce SLAM and other capabilities.
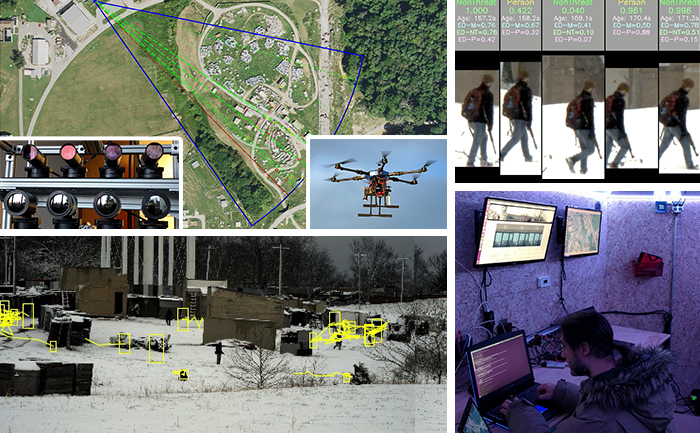
Cyber-Physical Systems
The physical environment presents a unique, ever-changing set of challenges to any sensing and analytics system. Kitware has designed and constructed state-of-the-art cyber-physical systems that perform onboard, autonomous processing to gather data and extract critical information. Computer vision and deep learning technology allow our sensing and analytics systems to overcome the challenges of a complex, dynamic environment. They are customized to solve real-world problems in aerial, ground, and underwater scenarios. These capabilities have been field-tested and proven successful in programs funded by R&D organizations such as DARPA, AFRL, and NOAA.
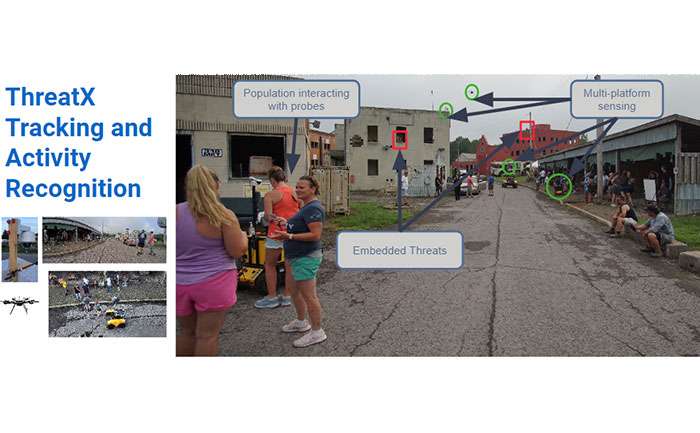
Complex Activity, Event, and Threat Detection
Kitware’s tools detect high-value events, behaviors, and anomalies by analyzing low-level actions and events in complex environments. Using data from sUAS, fixed security cameras, WAMI, FMV, MTI, and various sensing modalities such as acoustic, Electro-Optical (EO), and Infrared (IR), our algorithms recognize actions like picking up objects, vehicles starting/stopping, and complex interactions such as vehicle transfers. We leverage both traditional computer vision deep learning models and Vision-Language Models (VLMs) for enhanced scene understanding and context-aware activity recognition. For sUAS, our tools provide precise tracking and activity analysis, while for fixed security cameras, they monitor and alert on unauthorized access, loitering, and other suspicious behaviors. Efficient data search capabilities support rapid identification of threats in massive video streams, even with detection errors or missing data. This ensures reliable activity recognition across a variety of operational settings, from large areas to high-traffic zones.
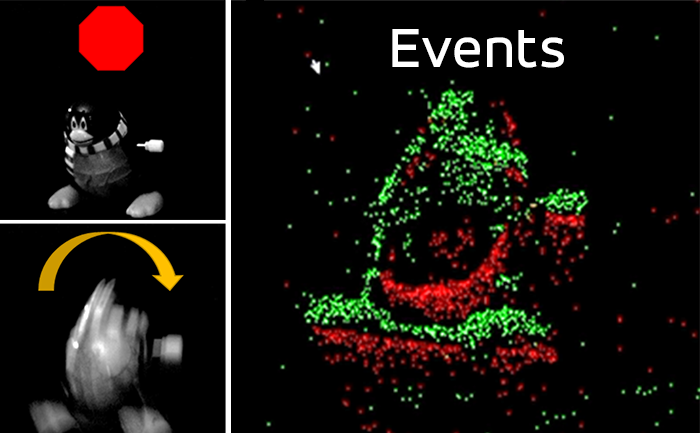
Computational Imaging
The success or failure of computer vision algorithms is often determined upstream, when images are captured with poor exposure or insufficient resolution that can negatively impact downstream detection, tracking, or recognition. Recognizing that an increasing share of imagery is consumed exclusively by software and may never be presented visually to a human viewer, computational imaging approaches co-optimize sensing and exploitation algorithms to achieve more efficient, effective outcomes than are possible with traditional cameras. Conceptually, this means thinking of the sensor as capturing a data structure from which downstream algorithms can extract meaningful information. Kitware’s customers in this growing area of emphasis include IARPA, AFRL, and MDA, for whom we’re mitigating atmospheric turbulence and performing recognition on unresolved targets for applications such as biometrics and missile detection.
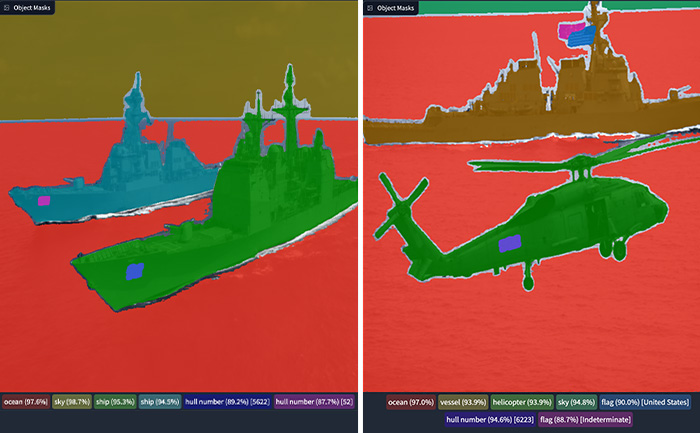
Generative AI
Through our extensive experience in AI and our early adoption of deep learning, we have made significant contributions to object detection, recognition, tracking, activity detection, semantic segmentation, and content-based retrieval for computer vision. With recent shifts in the field from predictive AI to generative AI (or genAI), we are leveraging new technologies such as large language models (LLMs) and multi-modal foundation models that operate on both visual and textual inputs. On the DARPA ITM program, we have developed sample-efficient methods to adapt LLMs for human-aligned decision-making in the medical triage domain.
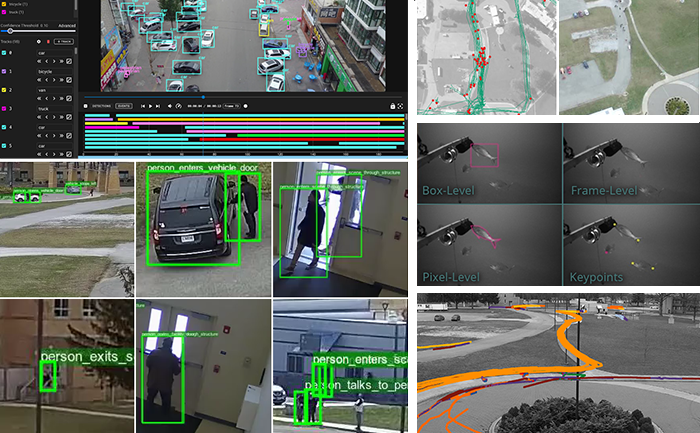
Dataset Collection and Annotation
The growth in deep learning has increased the demand for quality, labeled datasets needed to train models and algorithms. The power of these models and algorithms greatly depends on the quality of the training data available. Kitware has developed and cultivated dataset collection, annotation, and curation processes to build powerful capabilities that are unbiased and accurate, and not riddled with errors or false positives. Kitware can collect and source datasets and design custom annotation pipelines. We can annotate image, video, text and other data types using our in-house, professional annotators, some of whom have security clearances, or leverage third-party annotation resources when appropriate. Kitware also performs quality assurance that is driven by rigorous metrics to highlight when returns are diminishing. All of this data is managed by Kitware for continued use to the benefit of our customers, projects, and teams. Data collected or curated by Kitware includes the MEVA activity and MEVID person re-identification datasets, the VIRAT activity dataset, and the DARPA Invisible Headlights off-road autonomous vehicle navigation dataset.
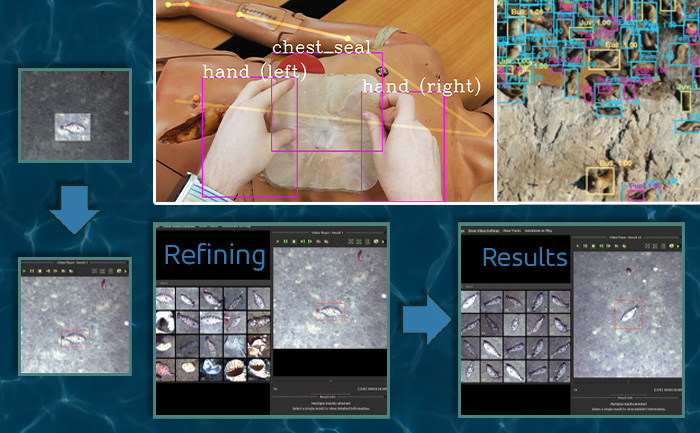
Interactive Artificial Intelligence and Human-Machine Teaming
DIY AI enables end users – analysts, operators, engineers – to rapidly build, test, and deploy novel AI solutions without having expertise in machine learning or even computer programming. Using Kitware’s interactive DIY AI toolkits, you can easily and efficiently train object classifiers using interactive query refinement, without drawing any bounding boxes. You are able to interactively improve existing capabilities to work optimally on your data for tasks such as object tracking, object detection, and event detection. Our toolkits also allow you to perform customized, highly specific searches of large image and video archives powered by cutting-edge AI methods. Currently, our DIY AI toolkits, such as VIAME, are used by scientists to analyze environmental images and video. Our defense-related versions are being used to address multiple domains and are provided with unlimited rights to the government. These toolkits enable long-term operational capabilities even as methods and technology evolve over time.
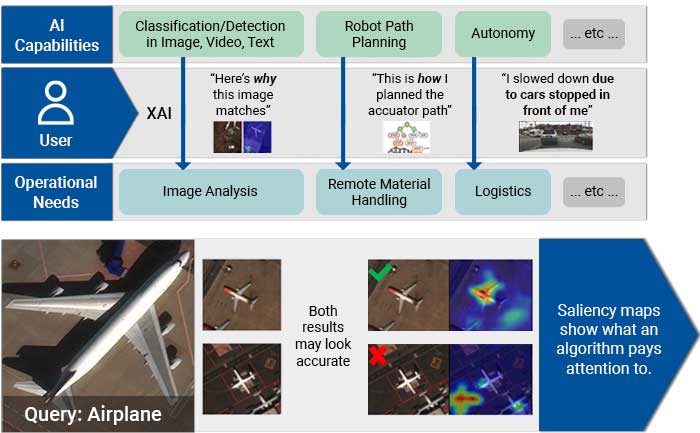
Explainable and Ethical AI
Integrating AI via human-machine teaming can greatly improve capabilities and competence as long as the team has a solid foundation of trust. To trust your AI partner, you must understand how the technology makes decisions and feel confident in those decisions. Kitware has developed powerful tools, such as the Explainable AI Toolkit (XAITK), to explore, quantify, and monitor the behavior of deep learning systems. Our team is also making deep neural networks more robust when faced with previously-unknown conditions, by leveraging AI test and evaluation (T&E) tools such as the Natural Robustness Toolkit (NRTK). In addition, our team is stepping outside of classic AI systems to address domain independent novelty identification, characterization, and adaptation to be able to acknowledge the introduction of unknowns. We also value the need to understand the ethical concerns, impacts, and risks of using AI. That’s why Kitware is developing methods to understand, formulate and test ethical reasoning algorithms for semi-autonomous applications. Kitware is proud to be part of the AISIC, a U.S. Department of Commerce Consortium dedicated to advancing the development and deployment of safe, trustworthy AI.
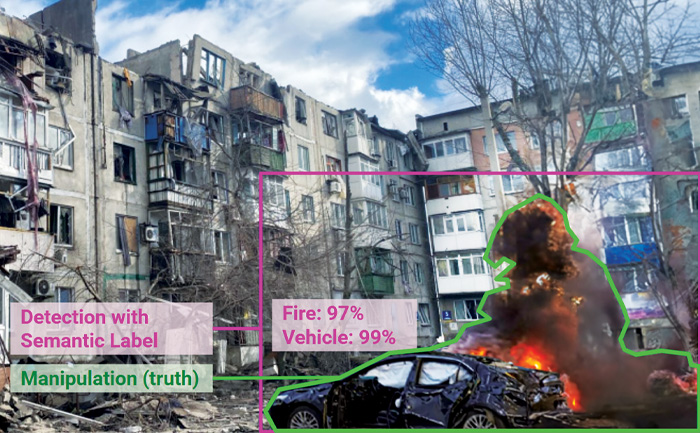
Combatting Disinformation
In the age of disinformation, it has become critical to validate the integrity and veracity of images, video, audio, and text sources. For instance, as photo-manipulation and photo-generation techniques are evolving rapidly, we continuously develop algorithms to detect, attribute, and characterize disinformation that can operate at scale on large data archives. These advanced AI algorithms allow us to detect inserted, removed, or altered objects, distinguish deep fakes from real images or videos, and identify deleted or inserted frames in videos in a way that exceeds human performance. We continue to extend this work through multiple government programs to detect manipulations in falsified media exploiting text, audio, images, and video.
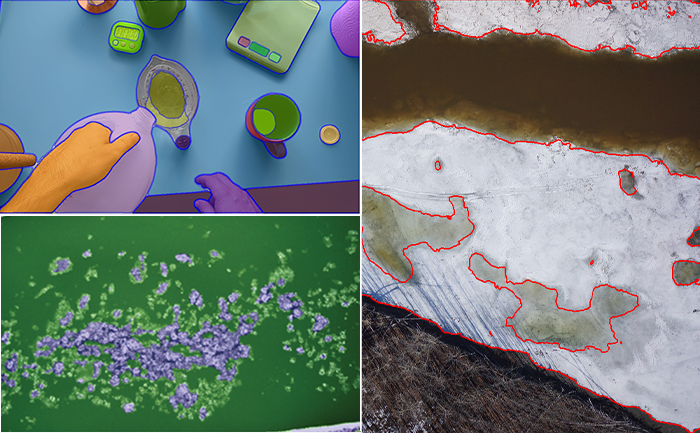
Semantic Segmentation
Kitware’s knowledge-driven scene understanding capabilities use deep learning techniques to accurately segment scenes into object types. In video, our unique approach defines objects by behavior, rather than appearance, so we can identify areas with similar behaviors. Through observing mover activity, our capabilities can segment a scene into functional object categories that may not be distinguishable by appearance alone. These capabilities are unsupervised so they automatically learn new functional categories without any manual annotations. Semantic scene understanding improves downstream capabilities such as threat detection, anomaly detection, change detection, 3D reconstruction, and more.
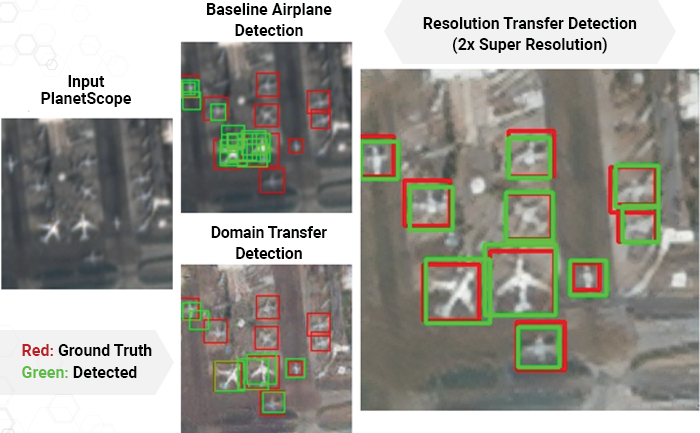
Super Resolution and Enhancement
Images and videos often come with unintended degradation – lens blur, sensor noise, environmental haze, compression artifacts, etc., or sometimes the relevant details are just beyond the resolution of the imagery. Kitware’s super-resolution techniques enhance single or multiple images to produce higher-resolution, improved images. We use novel methods to compensate for widely spaced views and illumination changes in overhead imagery, particulates and light attenuation in underwater imagery, and other challenges in a variety of domains. Our experience includes both powerful generative AI methods and simpler data-driven methods that avoid hallucination. The resulting higher-quality images enhance detail, enable advanced exploitation, and improve downstream automated analytics, such as object detection and classification.
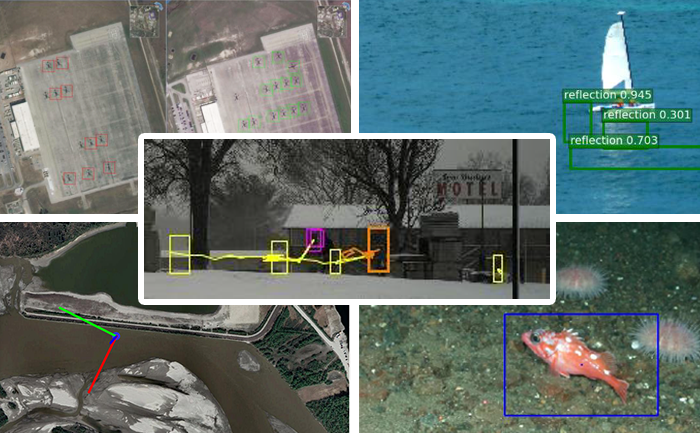
Object Detection and Classification
Our video object detection and tracking tools are the culmination of years of continuous government investment. Deployed operationally in various domains, our mature suite of trackers can identify and track moving objects in many types of intelligence, surveillance, and reconnaissance data (ISR), including video from ground cameras, aerial platforms, underwater vehicles, robots, and satellites. These tools are able to perform in challenging settings and address difficult factors, such as low contrast, low resolution, moving cameras, occlusions, shadows, and high traffic density, through multi-frame track initialization, track linking, reverse-time tracking, recurrent neural networks, and other techniques. Our trackers can perform difficult tasks including ground camera tracking in congested scenes, real-time multi-target tracking in full-field WAMI and OPIR, and tracking people in far-field, non-cooperative scenarios.
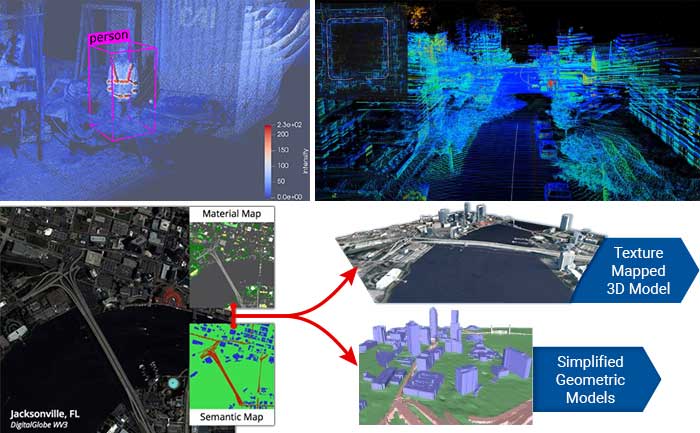
3D Reconstruction, Point Clouds, and Odometry
Kitware’s algorithms can extract 3D point clouds and surface meshes from video or images, without metadata or calibration information, or exploiting it when available. Operating on these 3D datasets or others from LiDAR and other depth sensors, our methods estimate scene semantics and 3D reconstruction jointly to maximize the accuracy of object classification, visual odometry, and 3D shape. Our open source 3D reconstruction toolkit, TeleSculptor, is continuously evolving to incorporate advancements to automatically analyze, visualize, and make measurements from images and video. LiDARView, another open source toolkit developed specifically for LiDAR data, performs 3D point cloud visualization and analysis in order to fuse data, techniques, and algorithms to produce SLAM and other capabilities.
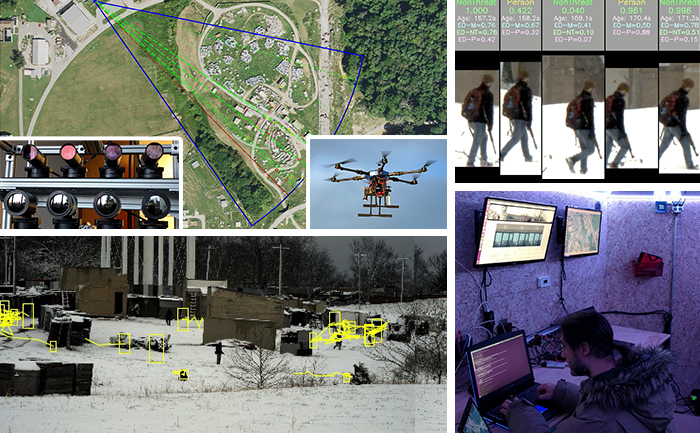
Cyber-Physical Systems
The physical environment presents a unique, ever-changing set of challenges to any sensing and analytics system. Kitware has designed and constructed state-of-the-art cyber-physical systems that perform onboard, autonomous processing to gather data and extract critical information. Computer vision and deep learning technology allow our sensing and analytics systems to overcome the challenges of a complex, dynamic environment. They are customized to solve real-world problems in aerial, ground, and underwater scenarios. These capabilities have been field-tested and proven successful in programs funded by R&D organizations such as DARPA, AFRL, and NOAA.
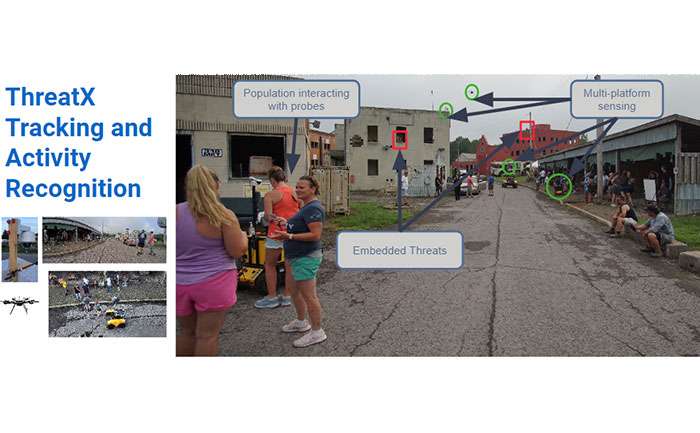
Complex Activity, Event, and Threat Detection
Kitware’s tools detect high-value events, behaviors, and anomalies by analyzing low-level actions and events in complex environments. Using data from sUAS, fixed security cameras, WAMI, FMV, MTI, and various sensing modalities such as acoustic, Electro-Optical (EO), and Infrared (IR), our algorithms recognize actions like picking up objects, vehicles starting/stopping, and complex interactions such as vehicle transfers. We leverage both traditional computer vision deep learning models and Vision-Language Models (VLMs) for enhanced scene understanding and context-aware activity recognition. For sUAS, our tools provide precise tracking and activity analysis, while for fixed security cameras, they monitor and alert on unauthorized access, loitering, and other suspicious behaviors. Efficient data search capabilities support rapid identification of threats in massive video streams, even with detection errors or missing data. This ensures reliable activity recognition across a variety of operational settings, from large areas to high-traffic zones.
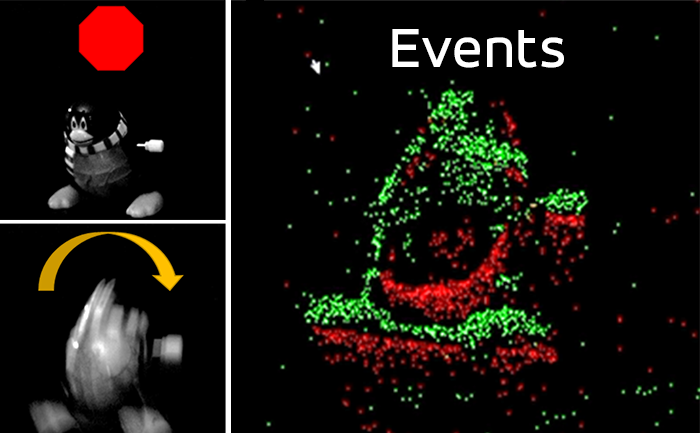
Computational Imaging
The success or failure of computer vision algorithms is often determined upstream, when images are captured with poor exposure or insufficient resolution that can negatively impact downstream detection, tracking, or recognition. Recognizing that an increasing share of imagery is consumed exclusively by software and may never be presented visually to a human viewer, computational imaging approaches co-optimize sensing and exploitation algorithms to achieve more efficient, effective outcomes than are possible with traditional cameras. Conceptually, this means thinking of the sensor as capturing a data structure from which downstream algorithms can extract meaningful information. Kitware’s customers in this growing area of emphasis include IARPA, AFRL, and MDA, for whom we’re mitigating atmospheric turbulence and performing recognition on unresolved targets for applications such as biometrics and missile detection.
Kitware’s Automated Image and Video Analysis Platforms
Interested in learning more? Let’s talk!
We are always looking for collaboration opportunities where we can apply our automated image and video analysis expertise. Let’s schedule a meeting to talk about your project and see if Kitware would be a good fit.