Advancing Undersea Soundscape Awareness: Kitware’s Maritime Acoustic Recognition and Identification with Novel Algorithms (MARINA) Project
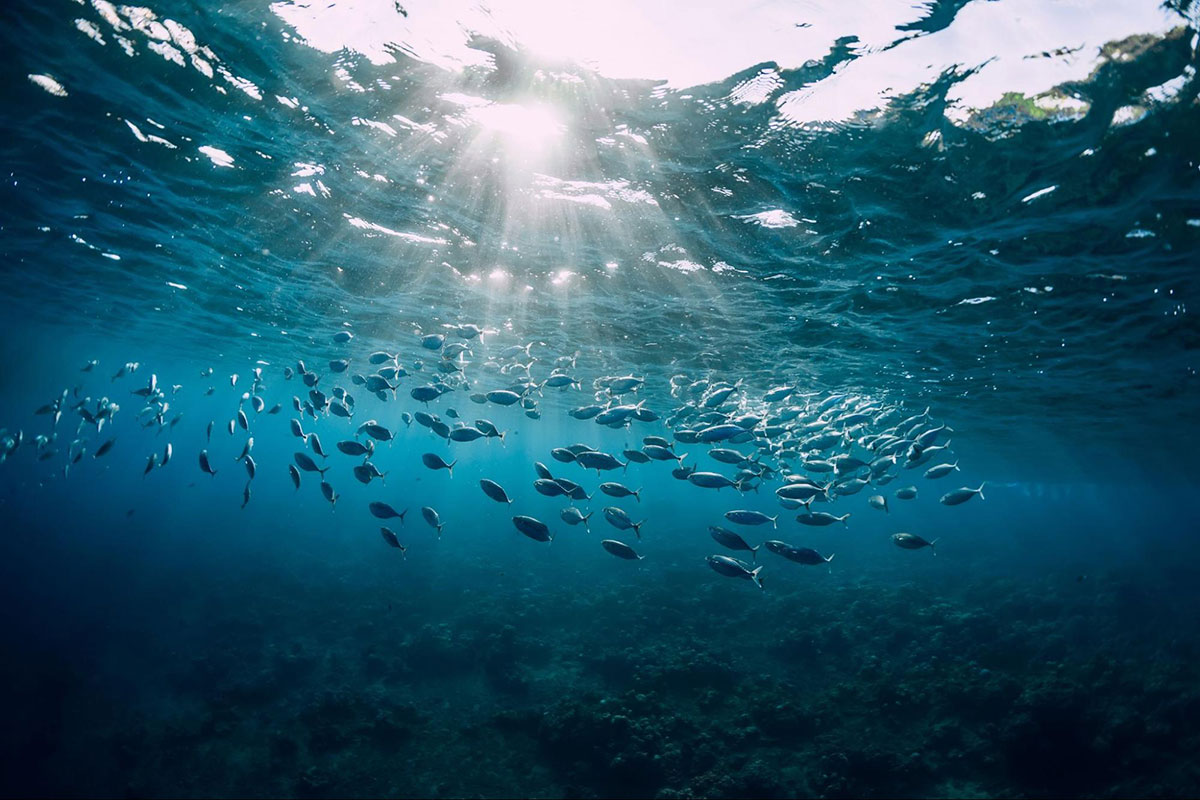
What is Underwater Soundscape Awareness?
The ocean’s acoustic environment is a complex tapestry of sounds originating from the natural environment (e.g. waves, currents), biological sources (e.g. marine mammals, fish), and humans (e.g. boats, drilling). Underwater soundscape awareness facilitates understanding and monitoring the acoustic environments in oceans and other bodies of water.
Why is this important? Marine animals depend on sound for essential behaviors. Any disruptions in underwater soundscapes, such as human-induced noise pollution, can significantly impact their communication, navigation, and survival.
The underwater domain also has strategic implications for national security, encompassing activities like submersible operations, safeguarding territorial waters, and monitoring potential threats. Effective monitoring and analysis of these underwater acoustic signals are vital for detecting and classifying objects or activities of interest, potentially providing early warnings of security threats or unauthorized activities. Furthermore, advancements in this area could aid in the development of more sophisticated underwater surveillance systems, enhancing a nation’s capability to protect its interests and maintain security in a largely unobservable and challenging environment.
A Challenging Environment
Undersea soundscape awareness presents several challenges due to the unique characteristics of the underwater environment and the complexity of acoustic signals. Sound propagation in water, behaving differently than in air, travels faster and over longer distances but is influenced by factors like temperature, salinity, depth, and physical barriers, adding complexity to underwater acoustic analysis. Furthermore, environmental factors such as water currents, temperature changes, and seasonal variations affect sound production and propagation, further complicating data interpretation. Lastly, data collection under the sea is logistically challenging and expensive, necessitating specialized equipment and often involving deployment in remote or deep-sea locations.
Successfully understanding underwater soundscapes necessitates interdisciplinary efforts, combining expertise from oceanography, acoustics, and machine learning. In recent years, deep learning (DL) has revolutionized data interpretation by achieving performance levels comparable to human experts. However, the application of these methods in the underwater environment has been impeded by several challenges. A primary challenge is the scarcity of labeled data, a critical requirement for deep learning models to function effectively. Another challenge is the oversimplified method of processing audio data in deep networks. Deep learning’s success in image classification has led to a common method of processing audio data, where sounds are converted into a visual format, typically spectrograms, which is an inherently lossy process. While this approach is convenient, it results in the loss of crucial acoustic information (e.g., amplitude and phase). Finally, uncrewed underwater vehicles (UUVs) and sonobuoys are a common platform for performing undersea sensing, but they provide only limited onboard computing ability due to the finite power available. In contrast, performant deep learning models are computationally expensive and difficult to deploy on low-SWaP (size, weight, and power) hardware commonly used in UUVs. Addressing these challenges is crucial for leveraging the full potential of DL in enhancing our understanding of underwater environments.
Addressing the Challenges of Undersea Soundscapes
Kitware is addressing these challenges through the MARINA project, funded by the Defense Advanced Research Projects Agency (DARPA). Our team is developing a set of AI foundation models that collect passive acoustic data from various platforms, from powerful topside systems to compact undersea drones. These models will help bootstrap Department of Defense stakeholders’ AI/ML solutions to better understand and monitor underwater environments.
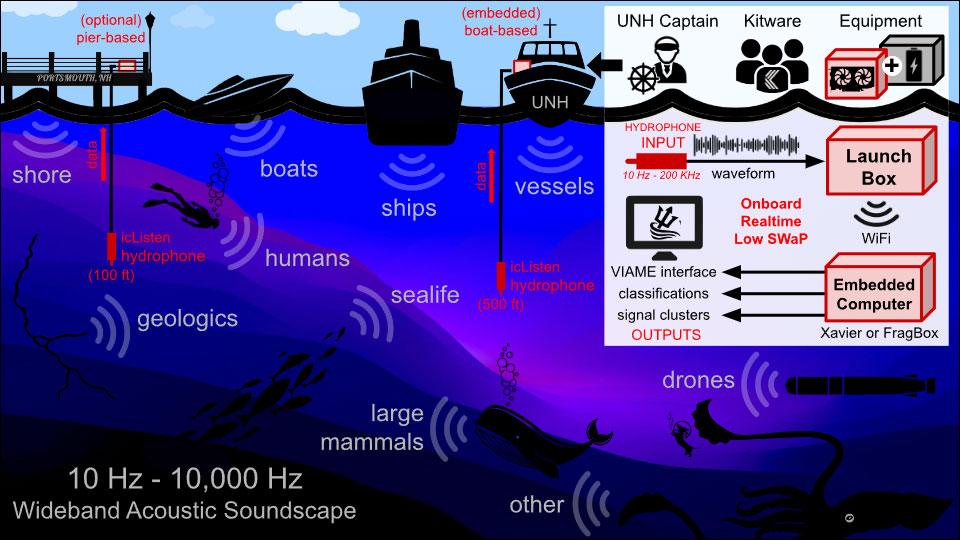
We are leveraging a hierarchical pretraining approach to take advantage of similarities between terrestrial and underwater acoustic patterns. Despite the different physical properties of sound in air versus water, common acoustic motifs exist in both environments. By using novel signal processing methods, we can adapt terrestrial sound patterns to match their underwater counterparts. This allows us to bootstrap the learning process using the abundant unlabeled terrestrial audio data, enabling effective low-shot learning for scarce underwater sound samples.
Unlike traditional spectrum-based approaches that process audio as images and discard phase information, our team is using advanced waveform analysis and data augmentation techniques. This allows us to preserve phase information which is known to be a discriminatory signal for some acoustic classes.
Due to the differing computing platforms, from powerful top-side computing systems to resource-constrained underwater vehicles (UUVs), we are developing and optimizing a range of foundation models. Using model distillation and pruning techniques, we created smaller, efficient versions of our models that maintain as much performance as possible while meeting strict size, weight, and power (SWaP) requirements across these diverse settings.
Our team is also expanding Kitware’s VIAME toolkit to handle acoustic data. This enhanced toolkit will enable users to classify sounds using established taxonomies and search for similar sounds within a database. To help users develop their own classifiers, the system will provide initial labels for user-submitted sounds, which can then be used to fine-tune deep learning models in real time. Additionally, we will add new visualization tools specifically designed for acoustic data analysis, making VIAME a comprehensive platform for both visual and acoustic data processing.
Expertise and Collaboration
This project combines two areas of expertise: Kitware’s capabilities in AI/ML and undersea signal processing and the University of New Hampshire’s knowledge of underwater acoustic sensing and marine mammal acoustics through Dr. Tom Blanford and Dr. Jennifer Miksis-Olds. To validate our acoustic soundscape analysis system, we will conduct several field tests using UNH’s research vessel, the R/V Gulf Challenger. During these tests, we will deploy hydrophones from the vessel to collect and analyze underwater sounds in real-time, demonstrating our system’s ability to classify and assess the underwater soundscape in real-time.
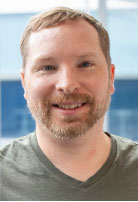
Dr. Isaac Gerg
Kitware
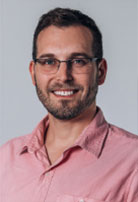
Dr. Jason Parham
Kitware
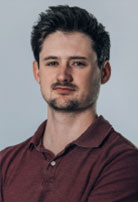
Dr. Albert Reed
Kitware
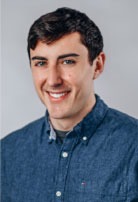
Dr. Connor Hashemi
Kitware
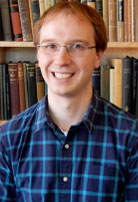
Dr. Tom Blanford
University of New Hampshire
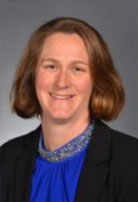
Dr. Jennifer Miksis-Olds
University of New Hampshire
Current Progress for MARINA
We’ve assembled over 1,800 hours of audio data from publicly available sources, including both underwater recordings and terrestrial acoustic datasets. Our unlabeled training data spans a diverse range of sounds: anthropogenic noise, bat and bird vocalizations, human speech, and various underwater acoustic signatures (e.g. biological, ambient, and ships). By utilizing the Department of Defense’s High-Performance Computing Modernization Program (HPCMP) Supercomputer, with almost 100 GPUs, we can efficiently process and learn from this extensive and diverse dataset.
A key advancement in our research is our novel approach to acoustic waveform analysis. Many existing systems convert acoustic waveforms to spectral representations (essentially treating them as images), discarding valuable phase information. Inspired by existing physical models derived from decades of past acoustic phenomenology research, our approach explicitly incorporates phase information without information loss, enabling more detailed, accurate downstream analytics such as fine-grained object recognition.
Applications and Impact
These foundation models will enhance undersea operations across multiple domains:
- Military defense systems
- Environmental monitoring
- Marine life conservation
- Underwater infrastructure monitoring
Contact our team to learn more about this developing technology.
The research reported in this document/presentation was performed in connection with contract number W912CG- 24-C-0020 with the U.S. Army Contracting Command – Aberdeen Proving Ground (ACC-APG) and the Defense Advanced Research Projects Agency (DARPA). The views and conclusions contained in this document/presentation are those of the authors and should not be interpreted as presenting the official policies or position, either expressed or implied, of ACC-APG, DARPA, or the U.S. Government unless so designated by other authorized documents. Citation of manufacturer’s or trade names does not constitute an official endorsement or approval of the use thereof. The U.S. Government is authorized to reproduce and distribute reprints for Government purposes notwithstanding any copyright notation hereon.